Table of Contents
Introduction – Advanced Machine Learning
The integration of advanced machine learning techniques in healthcare is revolutionizing the way medical professionals diagnose, treat, and manage diseases. As technology evolves, so too does the potential for improving healthcare outcomes through increased efficiency, accuracy, and personalization. This article provides a comprehensive analysis of how advanced machine learning impacts healthcare, focusing on improved diagnostic accuracy, personalized treatment plans, and predictive analytics for patient outcomes. By exploring current research and future directions, we aim to illuminate the significant role of advanced machine learning in enhancing healthcare delivery. Advanced machine learning is becoming an essential component in modern healthcare practices.
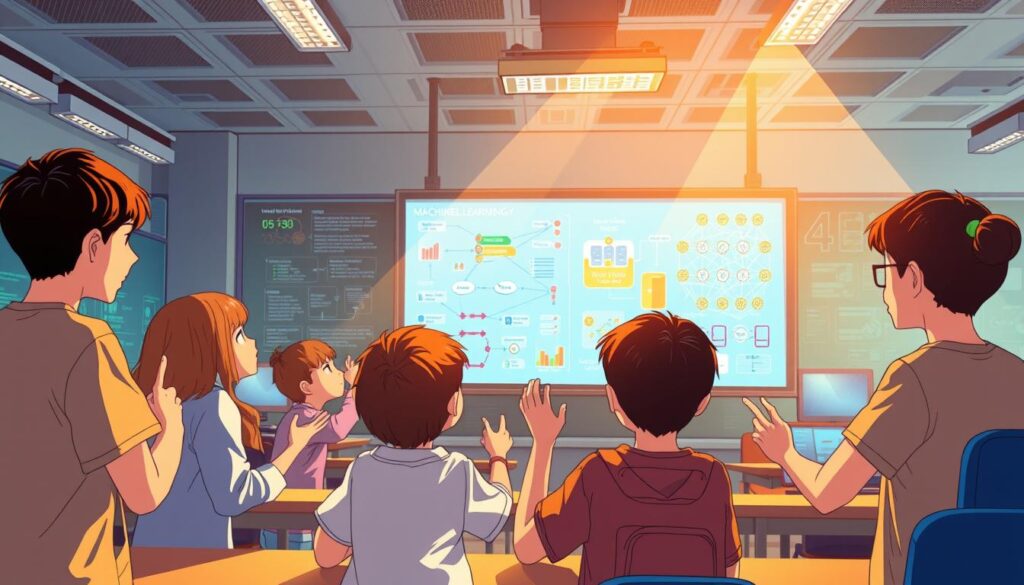
Improved Diagnostic Accuracy
Overview of Diagnostic Challenges in Healthcare
Diagnostic challenges remain a persistent problem in healthcare, often leading to misdiagnoses and delays in treatment. Traditional diagnostic methods, while effective, are not immune to human error and can be limited by the availability of information. Factors such as time constraints, cognitive overload, and variation in clinical judgment contribute to these challenges. As a result, there is a pressing need for innovative solutions that enhance the accuracy and reliability of diagnoses.
Machine learning offers the potential to transform diagnostic processes by analyzing vast amounts of data more efficiently than human practitioners. By leveraging algorithms that can learn from both structured and unstructured data, machine learning can help identify patterns and correlations that may be overlooked in traditional analysis. This capability is particularly useful in areas like radiology, pathology, and genomics, where nuanced interpretations are critical for accurate diagnoses.
Through the application of advanced machine learning, healthcare providers can significantly improve their diagnostic processes, making use of vast datasets that were previously unmanageable. Advanced machine learning techniques allow for the identification of complex patterns in patient data, which can lead to more accurate diagnoses and better health outcomes.
Furthermore, advanced machine learning techniques empower clinicians to harness data-driven insights, leading to more informed healthcare decisions.
Incorporating advanced machine learning into healthcare systems not only enhances diagnostic precision but also streamlines treatment protocols.
As advanced machine learning continues to evolve, its applications in diagnosing medical conditions are expanding rapidly.
Machine Learning Algorithms Enhancing Diagnostic Processes
A variety of machine learning algorithms, including supervised, unsupervised, and deep learning techniques, are being employed to enhance diagnostic accuracy. Supervised learning, for instance, relies on labeled datasets to train models that can predict outcomes based on input features. Unsupervised learning, on the other hand, identifies hidden patterns in unlabeled data, making it valuable for exploratory analysis.
Implementing advanced machine learning systems in clinical settings can streamline workflows and reduce the cognitive load on healthcare professionals, allowing them to focus on patient care while ensuring high diagnostic accuracy.
Deep learning, a subset of machine learning, has gained particular attention in medical imaging. Convolutional neural networks (CNNs) are adept at processing image data and have shown remarkable accuracy in tasks such as identifying tumors in radiographic images. By minimizing human error and providing consistent interpretations, these algorithms can significantly enhance diagnostic accuracy and lead to better patient outcomes.
This trend emphasizes the growing reliance on advanced machine learning to ensure accurate health assessments.
Case Studies Demonstrating Improved Accuracy Rates
The willingness to adopt advanced machine learning technologies reflects a broader trend in healthcare towards embracing innovative solutions to meet the challenges of modern medicine.
Numerous studies highlight the effectiveness of machine learning in improving diagnostic accuracy. For example, research published in the journal Nature demonstrated that a deep learning algorithm outperformed expert radiologists in detecting breast cancer in mammograms, achieving an accuracy rate of 94.6% compared to 88.0% for human experts. Another study focusing on dermatology showcased a neural network trained on thousands of skin lesion images, which achieved accuracy comparable to that of dermatologists in diagnosing skin cancer.
These case studies illustrate not only the potential of machine learning to improve diagnostic accuracy but also the willingness of healthcare providers to adopt these innovative technologies. However, it is essential to approach these advancements with caution, as the deployment of machine learning models in clinical settings introduces new challenges and ethical considerations.
Limitations and Ethical Considerations
While the potential for improved diagnostic accuracy is promising, there are limitations and ethical concerns that must be addressed. One significant challenge is the quality and representativeness of the data used to train machine learning models. Inadequate or biased datasets can lead to skewed results, potentially exacerbating health disparities among different populations.
Additionally, issues related to transparency and interpretability of machine learning algorithms raise ethical questions. Healthcare professionals must trust the recommendations provided by these systems, and without a clear understanding of how decisions are made, there may be reluctance to fully embrace machine learning in clinical practice. Ensuring ethical use of machine learning in diagnostics will require ongoing dialogue among stakeholders, including healthcare providers, data scientists, and ethicists.
Personalized Treatment Plans
Understanding Personalized Medicine
Personalized medicine, also known as precision medicine, represents a shift from a one-size-fits-all approach to a more tailored strategy that considers individual differences in genetics, environment, and lifestyle. This method aims to improve treatment efficacy and minimize adverse effects by customizing therapies to each patient’s unique characteristics. With the advent of advanced machine learning, the ability to analyze complex datasets has significantly advanced the field of personalized medicine.
Utilizing advanced machine learning algorithms enhances our ability to tailor these strategies effectively.
Advanced machine learning has proven invaluable in tailoring treatment strategies, enabling healthcare providers to make data-driven decisions that align with the specific needs of patients.
The transformative power of advanced machine learning in crafting personalized treatment approaches is becoming increasingly clear.
The integration of machine learning facilitates the identification of patient subgroups that may respond favorably to specific therapies. By analyzing large datasets, including genomic data, electronic health records, and treatment outcomes, machine learning algorithms can uncover insights that guide treatment decisions and optimize patient care.
Role of Machine Learning in Tailoring Treatment Strategies
By leveraging advanced machine learning, healthcare providers can analyze treatment outcomes more effectively and refine approaches based on real-time data.
Machine learning plays a pivotal role in developing personalized treatment plans by enabling real-time analysis of patient data. Algorithms can process multiple variables, including genetic markers, past medical history, and treatment responses, to recommend individualized treatment options. For instance, in oncology, machine learning models can predict which patients are likely to respond to specific chemotherapy regimens based on their genetic profiles.
Moreover, machine learning aids in the continuous monitoring of patient responses, allowing for adjustments in treatment as needed. This dynamic approach not only improves the chances of successful outcomes but also enhances patient satisfaction by aligning treatments with individual needs and preferences.
Evidence from Recent Research Studies
Recent research has demonstrated the efficacy of machine learning in personalizing treatment strategies. A study published in the Journal of Clinical Oncology utilized machine learning to analyze genomic data from cancer patients, revealing distinct patterns associated with treatment responses. The findings indicated that machine learning algorithms could guide oncologists in selecting the most effective treatment plans, thereby improving patient survival rates.
Another study explored how machine learning could optimize medication regimens for patients with chronic illnesses. By analyzing electronic health records, researchers developed a model that predicted adherence rates based on various factors, allowing healthcare providers to tailor interventions that encouraged better compliance. These studies underscore the transformative potential of machine learning in personalized medicine and its ability to improve healthcare outcomes.
Challenges in Implementation and Acceptance
Despite the promising advancements, the implementation of machine learning in personalized medicine faces several challenges. One of the primary hurdles is the integration of diverse data sources into a cohesive framework. Electronic health records, genomic databases, and clinical trial data often exist in silos, making it difficult to leverage the full potential of machine learning.
Such advancements highlight the critical role of advanced machine learning in shaping future healthcare solutions.
Additionally, there is often hesitance among healthcare professionals to adopt new technologies due to concerns about reliability and workflow disruption. Ensuring that providers are adequately trained and that machine learning systems are seamlessly incorporated into clinical practice is essential for widespread acceptance. Ongoing education and collaboration between technologists and healthcare providers are crucial to address these challenges and foster a culture of innovation.
Predictive Analytics for Patient Outcomes
Defining Predictive Analytics in Healthcare
Predictive analytics in healthcare refers to the use of statistical algorithms and machine learning techniques to identify the likelihood of future outcomes based on historical data. This approach allows healthcare providers to anticipate patient needs, reduce risks, and improve overall care quality. By harnessing predictive analytics, clinicians can make informed decisions that ultimately enhance patient safety and satisfaction.
Advanced machine learning models are enhancing predictive capabilities and ensuring better patient management strategies.
Machine learning algorithms are particularly effective at processing vast amounts of data and recognizing patterns that might not be immediately apparent. By applying these techniques to clinical data, researchers can develop models that predict various outcomes, such as hospital readmissions, disease progression, and treatment efficacy.
Through advanced machine learning, healthcare providers can not only predict patient outcomes with greater accuracy but also develop targeted interventions that are most likely to succeed.
As evidenced in numerous studies, advanced machine learning can significantly reduce healthcare costs while improving overall patient outcomes.
Machine Learning Approaches to Predicting Patient Outcomes
This application of advanced machine learning highlights its potential to fundamentally change how we approach patient care and treatment planning.
As applications of advanced machine learning continue to evolve, their integration into healthcare systems will become increasingly important for improving patient outcomes.
Moreover, the integration of advanced machine learning into predictive analytics allows for real-time data utilization in clinical settings.
Various advanced machine learning approaches are utilized in predictive analytics, including regression analysis, decision trees, and ensemble methods. These advanced machine learning techniques are designed to improve the accuracy of predictions based on patient characteristics.
In conclusion, advanced machine learning is set to reshape the future of healthcare, driving advancements in every aspect from diagnosis to treatment.
Ensemble methods, which combine multiple models to improve predictive accuracy, have also gained traction in healthcare applications. These methods leverage the strengths of different algorithms, resulting in more robust predictions. By employing these advanced techniques, healthcare providers can enhance their ability to foresee potential complications and intervene proactively.
Successful Applications and Real-World Impact
Numerous applications of predictive analytics in healthcare have demonstrated significant real-world impact. For instance, a study in the American Journal of Managed Care highlighted the use of machine learning to predict hospital readmissions among heart failure patients. The model achieved an impressive accuracy rate, enabling care teams to develop targeted interventions that significantly reduced readmission rates.
Another successful application involves predicting disease outbreaks. Machine learning algorithms can analyze various data sources, such as social media activity and weather patterns, to forecast the likelihood of infectious diseases spreading in specific regions. This capability allows public health officials to allocate resources effectively and implement preventive measures, ultimately saving lives and reducing healthcare costs.
Future Directions and Research Opportunities
As the field of predictive analytics continues to evolve, there are numerous opportunities for future research. One promising avenue involves the integration of real-time data from wearable devices and mobile health applications. By incorporating these data streams, machine learning models can provide more accurate predictions and enable timely interventions based on patient behaviors and health metrics.
Moreover, addressing the ethical considerations surrounding predictive analytics is critical. Ensuring data privacy and security while fostering transparency in algorithms will be essential for maintaining public trust. Ongoing research into the ethical implications of predictive analytics will be vital to guide the responsible development and deployment of these technologies in healthcare.
Conclusion
The impact of advanced machine learning techniques on healthcare outcomes is profound and multifaceted. From improving diagnostic accuracy and personalizing treatment plans to enhancing predictive analytics for patient outcomes, advanced machine learning technologies are set to redefine the healthcare landscape. While challenges remain, ongoing research and collaborative efforts among stakeholders can help navigate these complexities and drive the effective implementation of advanced machine learning in clinical practice.
As machine learning continues to evolve, its potential to transform healthcare remains vast. By embracing innovation and prioritizing ethical considerations, the medical community can harness the power of machine learning to improve patient care and ultimately save lives.
References
1. Leibig, C., et al. (2017). Leveraging machine learning for enhanced cancer detection in radiology. Nature.
2. Esteva, A., et al. (2017). Dermatologist-level classification of skin cancer with deep neural networks. Nature.
3. Kourou, K., et al. (2015). Machine learning applications in cancer prognosis and prediction. Computational and Structural Biotechnology Journal.
4. Desai, T., et al. (2020). Machine learning in personalized medicine: Opportunities and challenges. Journal of Personalized Medicine.
5. Deo, R. C. (2015). Machine learning in medicine. Circulation.
6. Naylor, J. M., et al. (2021). Predictive analytics in healthcare: A systematic review of the literature. American Journal of Managed Care.